Machine learning algorithm allows for multi-institution collaboration without sharing data
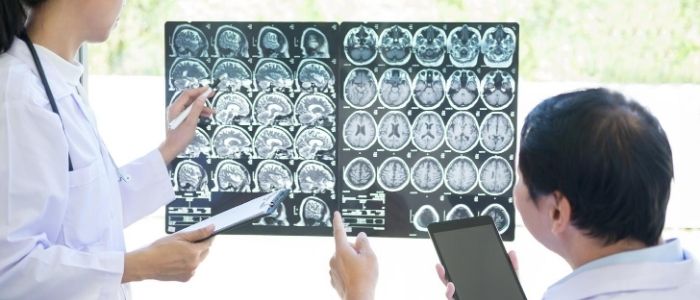
Researchers have successfully used a machine learning technique, known as federated learning, to create a multi-institution trained model that could help clinicians make decisions related to brain imaging.
Studies have demonstrated the power of machine learning in medicine, highlighting its ability to identify complex patterns and provide answers to complex questions. However, the issue with machine learning models is that they require large amounts of patient data, which institutions and hospitals are often unable to share due to patient confidentiality.
Using an emerging technique, known as federated learning, a multi-institutional team of researchers may have overcome this issue through the development of a machine learning model that does not rely on the sharing of private patient data.
The technique, as described in a recent article published in Scientific Reports, involves each data-owner training the algorithm separately. The results are then aggregated so that the model is trained on all data without the data being shared between the different institutions.
Although the approach would be useful in many medical settings, the researchers focused on its applications in brain imaging for this study. Having doctors around the world add brain scan images to a decentralized model would allow for the development of a consensus model that can analyze brain MRI scans and distinguish between healthy brain tissue and cancerous regions.
Anne Carpenter on artificial intelligence in the cell imaging field
At ASCB 2019, BioTechniques sat down with the Broad Institute’s Anne Carpenter to discuss her work in cell imaging software and how artificial intelligence is revolutionizing the field.
“The more data the computational model sees, the better it learns the problem, and the better it can address the question that it was designed to answer,” remarked senior author Spyridon Bakas (University of Pennsylvania, PA, USA). “Traditionally, machine learning has used data from a single institution, and then it became apparent that those models do not perform or generalize well on data from other institutions.”
In order to compare the effectiveness of federated learning against other machine learning models, the team investigated a model pre-trained on multi-institutional data from the International Brain Tumor Segmentation Challenge, a dataset containing more than 2600 brain scans from 660 patients. Ten hospitals also trained models with their own patient data. Federated learning was used to create a consensus model from all of this data.
The researchers then compared the federated learning model to other machine learning models trained by both single institutions and collaboratively by measuring their effectiveness against scans manually annotated by neurologists. They found that federated learning was the most effective and that increasing access to data through collaborations can benefit model performance.
These findings have inspired a much larger, international study in which 30 different institutions across 9 countries will use federated learning to train a consensus model on brain tumor data, hopefully resulting in the production of an open-source tool any clinician, at any hospital, can use to make decisions about brain tumor patient care.
“Studies have shown that, when it comes to tumor boundaries, not only can different physicians have different opinions, but the same physician assessing the same scan can see different tumor boundary definitions on one day of the week versus the next,” Bakas explained. “Artificial Intelligence allows a physician to have more precise information about where a tumor ends, which directly affects a patient’s treatment and prognosis.”