Pick of the posters: AACR 2025
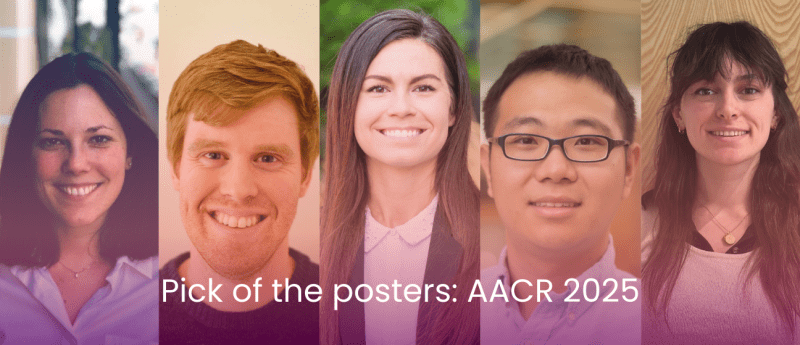
At this year’s AACR 2025 (Chicago, IL, USA; 25–30 April), the annual meeting of the American Association for Cancer Research, we picked out our favourite posters from the conference and spoke to their presenters to get the inside line on the techniques used and the approaches taken.
Contents:
AI driven discovery of novel synthetic lethal gene pairs for targeted cancer therapy
Bioprinting 3D spatially resolved tumor avatars to mimic the native tumor microenvironment
Intratumoral bacteria predict survival in clear cell renal cell carcinoma
AI driven discovery of novel synthetic lethal gene pairs for targeted cancer therapy
Daniel Miller,
Director of Biology at Evariste (London, UK)
Amalia Soenens,
Senior Product Manager at EditCo Bio (CA, USA)
Please describe the data presented in your poster and its key takeaways
We used Evariste’s AI-driven target identification platform to discover the next generation of synthetic lethal gene targets and then collaborated with EditCo to validate these on a large scale by generating 72 CRISPR knockout cell pools of 24 unique biomarkers across three cell lines simultaneously. To demonstrate the advantages of this approach, we identified a novel synthetic lethal relationship for the known cancer target FAK, engineered highly selective small molecule inhibitors and further validated the synthetic lethal biomarker. Taken together, this data shows the power of combining AI modeling with high-throughput cell engineering to rapidly discover and validate new drug targets with high confidence.
What techniques were used in the generation of this data?
We used AI modeling – a suite of proprietary AI tools that ingest and harmonize large biological datasets, including multiomics, clinical data and cancer dependency data, analyze these datasets for high-confidence synthetic lethal relationships and output novel druggable targets. Evariste’s advanced AI-driven Frobenius Target platform allowed us to screen 400 million putative synthetic pairs in silico and narrow to 24 potential novel synthetic lethals we could validate in vitro through CRISPR editing.
We also used gene editing – CRISPR knockout models were generated with EditCo’s XDel technology in combination with our high-throughput gene editing platform. XDel Knockout Cells outperform traditional single-guide RNA (sgRNA) CRISPR methods through a cooperative multi-guide RNA design, resulting in a fragment deletion targeted at a single exon and delivering significantly higher on-target editing efficiency while minimizing off-target effects. This approach ensures persistent protein depletion and eliminates the variability often encountered with traditional CRISPR methods.
Do you have any tips for using these techniques?
One of the biggest tips we can offer is to work with a partner who can simplify and accelerate the discovery process, especially when you’re focused on functional genomics or cell-based assays. Leveraging an advanced and end-to-end CRISPR platform provides a robust and scalable process to take the complexity out of edited cell-model generation. This allows researchers to spend less time troubleshooting and more time generating data.
CRISPR has transformed how scientists study gene function, but it still presents challenges, from design to optimization to delivery and validation. The goal is to give researchers reliable tools they can trust, with high-quality cellular models supporting confidence in downstream assays.
What impact do you hope this poster will have on cancer research?
Too few cancer patients receive a precision oncology treatment. By developing tools that accelerate the identification of novel drug targets with robust biomarkers, we will allow the development of a wider range of therapeutic options so that patients can get effective treatments with fewer side effects. By improving on and scaling up technologies like AI and CRISPR, we can find these targets, test novel therapies, and move cancer research forward faster and more efficiently than has been possible in the past.
Bioprinting 3D spatially resolved tumor avatars to mimic the native tumor microenvironment
Haylie Helms
PhD Student, Oregon Health and Science University (OR, USA)
Can you introduce your poster and the method that it presents?
My work focuses on making in vitro models where we can control what cell types are present and their spatial organization. We can ask questions about density, distance and more. My prior work was making 2D replicas where we would take a patient biopsy that has been annotated, look at where each of those cells are, grow them in culture, isolate each cell and put them down one by one with 1.6 micron resolution.
We wanted to expand that work into 3D, so now we’re taking the patient biopsy again and using it to create an annotated map of cell types. This map is not quite at single-cell resolution; instead, we look at neighborhoods like the stromal compartment or the cancer compartment. In the example featured in my poster, you can see seven different cell types in one of these maps.
We then use commercially available primary cells or cells taken from the patient biopsy to 3D print a replica or ‘tumor avatar’ with each of those different cell types deposited into their respective habitats. The poster then shows that when we overlay the map of the patient’s biopsy on top of the 3D tumor avatar, you can see it’s to scale and physiologically comparable; it has the same immune cells, the duct shapes are the same and are also full of cancer, it’s amazing.
What differentiates these bioprinted tumor replicas from a traditional tumor organoid?
The power of this platform is that you get to control which cells are present, where they are located and their geometry. In conventional spheroids or organoids, you can put the same number of cells and ratios, but then you rely on the cells to self-organize, instead of being able to control where those cell types are.
Do you have any tips for using this kind of technique?
This is still in development. But once the method is out, it should be very user-friendly: you grow out your cells, load the different cell types into the printer, move the print head over the area of the organoid you want them to go and dispense them out.
What would you hope the impact of this research or this method will be on cancer research?
I hope this technology will be applicable to any type of cancer, to allow researchers to controllably and reproducibly ask lots of questions and to perform wide range of experiments, such as mechanistic or drug studies. I see this as a tool that will complement in vivo studies, organoid models, and all the existing tools that we have, rather than replacing any of them. This new control of spatial organization could be game changing, for example, if you’re looking at patient biopsies and you observe an interesting interaction, but you want to know what would have happened if that tumor had continued to grow, you could make a replica, grow it over time and then see how it evolves.
Intratumoral bacteria predict survival in clear cell renal cell carcinoma
Alice Martin
PhD student, Francis Crick Institute (London, UK)
Please describe the data presented in your poster and its key takeaways.
In my poster, I presented my work on the intratumoral microbiome in clear cell renal cell carcinoma (ccRCC). We’ve discovered that Cutibacterium is the most abundant bacterial genus in ccRCC tumors and that its presence is associated with worse patient survival and immunotherapy resistance, thereby establishing it as a potential biomarker to improve patient stratification for adjuvant therapy administration.
Cutibacterium may drive disease progression by inducing pro-tumorigenic myeloid cell changes likely mediated via endothelial cell remodeling and neo-angiogenesis.
We are now running co-culture experiments to assess whether Cutibacterium plays an active role in remodeling the tumor microenvironment as well as building on our in situ imaging to identify the exact cell types that the bacteria infect or accumulate close to.
What techniques were used to generate this data?
We extracted bacterial reads from whole-genome sequencing and bulk RNAseq using a series of tools to ensure strict denoising and decontamination of the data. We worked with collaborators to culture bacterial colonies of Cutibacterium from tumor samples. We also used RNAscope on patient tumors to visualize Cutibacterium inside the tumors.
Do you have any tips for using these techniques?
When extracting bacterial reads from whole-genome sequencing data, careful denoising and decontamination is required. Several papers have been published discussing the pitfalls to avoid, with the key takeaways being:
- Avoid mismapping of human reads by including a human reference genome at every step and mapping final bacterial calls back to the T2T genome to ensure they do not stem from badly resolved areas of the human genome
- Remove common lab contaminants by sequencing water samples that you have taken through the same extraction protocol
- Confirm live presence of bacteria through culture.
For visualizing the bacteria with RNAscope, fresh frozen tissue gave us much better signal/noise quality to pick up this sparse signal than fixed tissue.
What impact do you hope this poster will have on cancer research?
This work lays the foundation for establishing intratumoral bacteria as novel targets, the elimination or modulation of which could ultimately act to enhance outcomes, reduce immunotherapy resistance and extend survival for patients with advanced ccRCC.
Visium HD combined with deep-learning-based cell segmentation on H&E images yield accurate cell annotation at single-cell resolution
Tianyou Luo
Machine Learning Scientist at Tempus AI (IL, USA).
Please describe the data presented in your poster and its key takeaways.
In this poster, we successfully mapped 2 𝜇m-resolution Visium HD data to single-cell gene counts in non-small cell lung cancer (NSCLC) samples by utilizing a trained neural network for cell segmentation on H&E-stained images. The resulting cell clusters, annotated using a large language model (LLM), showed a high degree of concordance with pathologist annotations, accurately identifying lymphocytes, cancer cells, and benign epithelium.
This approach enhances the potential for precise clinical biomarker discovery with high-resolution, whole transcriptome spatial transcriptomics and a deeper understanding of the TME.
Please tell us more about the method presented, how does it improve on existing approaches?
Spatial transcriptomics has emerged as an exciting field in recent years, especially with the launch of Visium HD platform from 10X Genomics, which enables whole-transcriptome spatial profiling at near sub-cellular resolution. However, the default binning of Visium HD at 8 𝜇m resolution overlooks cell morphology and complicates downstream biological interpretations of the data by merging multiple cells in one bin or splitting large cells into multiple bins.
Our approach addresses that challenge and demonstrates the feasibility and advantages of analyzing Visium HD data at single-cell resolution. This allows for applying standard single-cell RNA-seq analysis techniques for downstream biomarker discovery and achieves enhanced biological interpretability compared to default 8 𝜇m pixel resolution.
Do you have any tips for using this method?
Successful cell-level binning relies on high-quality nuclei segmentations, which necessitates a high-quality H&E image free of significant artifacts. It is also important to keep in mind the inherent data sparsity associated with Visium HD data when attempting to apply standard single-cell RNA-seq analysis toolboxes for downstream applications.
What impact do you hope this poster will have on cancer research
NGS-based spatial transcriptomics technologies have gained increasing popularity for their ability to provide spatial context of gene expression and investigate TMEs. I hope our work in this poster will help enhance our understanding of the TME at the single-cell level, thereby empowering further clinical biomarker discovery and offering new mechanistic insights for immuno-oncology and antibody-drug conjugates (ADCs).