GelGenie: magicking gel electrophoresis analysis into the 21st century
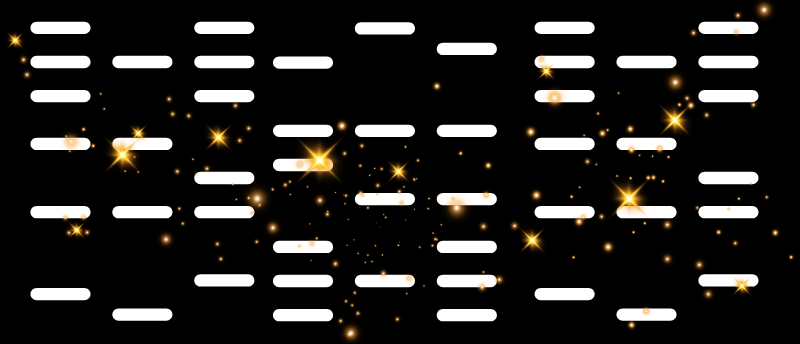
A new machine learning model can successfully identify bands from gel electrophoresis images.
Gel electrophoresis is a staple of many life science labs but has changed little since the 20th century. Now, researchers from the University of Edinburgh (UK) have developed a freely available machine learning model that can speed up the analysis of gel electrophoresis images.
Gel electrophoresis is widely used throughout the life sciences to separate and analyze biomolecules. While computational approaches have revolutionized image and data processing in many fields, the analysis of gel images has remained essentially unchanged for decades.
Most gel electrophoresis image analysis approaches involve digitally carving out lanes and bands from an image before summing the intensity of the pixels in each band. This process is performed either manually or semi-automatically, making it tedious and prone to human error. Additionally, it can be challenging to address bands with irregular shapes or curved trajectories.
Now you see me: machine learning model makes spectra clearer
A machine learning model has been developed that makes optical spectroscopy data easier and quicker to interpret.
The team investigated whether a machine learning tool could be developed to improve current gel image analysis workflows. They trained a lightweight neural network to identify bands from images, using a dataset of over 500 human-labeled gel images featuring a range of common experimental scenarios. The resulting model could successfully identify bands regardless of their quality, background intensity and even the presence of unexpected discontinuities such as torn gel chunks. The approach could even produce quantitative results that matched or surpassed those generated using conventional tools.
The team then released their models through GelGenie, an open-source application that allows users to extract bands from gel images on their own devices, with no expert knowledge or experience required.
“To the best of our knowledge, GelGenie is the first software platform to investigate universal gel analysis using AI,” commented Matthew Aquilina, who is now a research fellow at Harvard University and the Dana-Farber Cancer Institute (both MA, USA). “We hope our platform has set the stage for a truly universal gel analysis framework that others will integrate into their workflow and continue to iterate on with further refinements and improved functionality.”