Now you see me: machine learning model makes spectra clearer
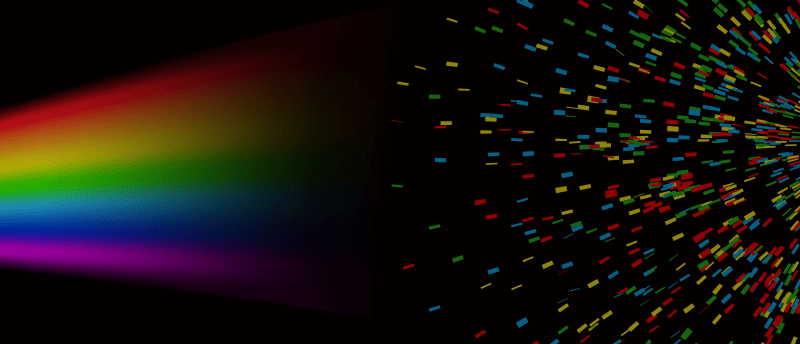
A machine learning model has been developed that makes optical spectroscopy data easier and quicker to interpret.
Researchers from Rice University (TX, USA) have developed a new machine learning algorithm that interprets optical spectra of molecules, materials and disease biomarkers in biological samples, including fluid samples containing SARS-CoV-2 spike protein. The model could be used to improve diagnostics and sample analysis.
Optical spectroscopy is used to determine the physical, chemical or structural properties of a sample by shining a laser onto the material and observing how light interacts with it. While a useful and widely utilized technique, interpreting the resulting spectral data can be challenging and time-consuming, especially when the differences between samples are subtle.
To address this, the researchers developed a machine learning algorithm called Peak-Sensitive Elastic-net Logistic Regression (PSE-LR) that is tailored for spectral analysis. PSE-LR classifies samples and produces a peak-sensitive feature importance map, which highlights the parts of the spectrum that contributed to the classification decision, making results easier to interpret, verify and act on.
Our top picks for SLAS Europe 2025
The Society for Laboratory Automation and Screening (SLAS) annual European conference and exhibition will be uniting the life sciences and laboratory technology community in Hamburg (Germany) from 20–22 May.
The researchers compared their model to other existing ones and found that it performed better, particularly when identifying subtle or overlapping spectral features. “Most models either miss the tiny details or are too complex to understand,” first author Ziyang Wang said. “We aimed to fix that by building something both smart and explainable.”
When applied to real-world samples, the model successfully detected ultralow concentrations of the SARS-CoV-2 spike protein in fluid samples, identified neuroprotective solutions in mouse brain tissue, classified Alzheimer’s disease samples and distinguished between 2D semiconductors.
The model could help develop new diagnostics, biosensors or nanodevices. “These findings could help transform medical diagnostics and materials science, bringing us closer to a world where smart technologies help detect and respond to health problems faster and more effectively,” concluded Wang.