COVID-19 retractions put the spotlight on bad data
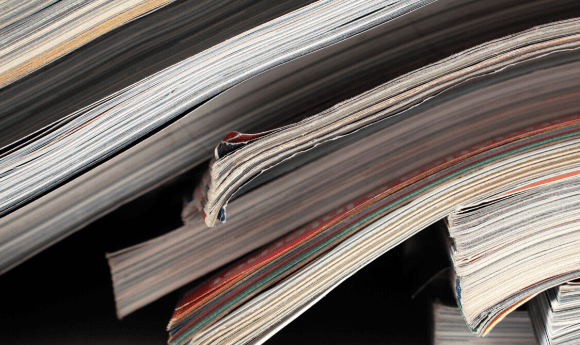
The recent news regarding the hydroxychloroquine and COVID-19 retractions highlights data remains a problem for scientific integrity and reproducibility.
The announcement of the retraction of two COVID-19 studies owing to false data has highlighted two issues – first, that while it is critical we endeavor to publish scientific research as soon as possible, our haste could be leading to mistakes; and second, that datasets that are analyzed in but not created as part of a study need more oversight. Alone, each issue can damage science; in combination, they could endanger lives.
If you would like to keep up to date with our content on coronavirus, you can sign up for our site here, where you can subscribe to our newsletters for free!
The two studies in question utilized a database from Surgisphere – a healthcare analytics company whose CEO, Sepan Desai, was a co-author on both papers – which purported to collect anonymized patient information.
For the first, published in The Lancet, it came to light post-publication that while the Surgisphere data provided records of 600 Australian COVID-19 patients and 73 deaths as of 21st April 2020, in reality, the number of deaths did not rise to 73 until the 23rd April. After some wrangling, the three other authors (that is, not Desai) retracted it as they were unable to complete an audit of the data [1,2]. The results of this study had had a large impact – the group found that hydroxychloroquine was associated with a higher COVID-19 mortality rate and an increase in heart problems. This led the WHO to halt a trial, although this then resumed once the initial expression of concern was published [3].
For the New England Journal of Medicine retraction, Desai agreed – the retraction notice states that not all authors were granted access to the raw data nor could it be audited by a third-party, hence the retraction [4].
It is unclear whether the rush to publish these seemingly important results led to these mistakes, for example through over-hasty peer review leading the reviewers to miss the data errors – if the underlying data were even available to them. What is clear is that the data source had too little oversight, and this brings into question whether rules surrounding data creation and validation need to be changed.
False negatives: how accurate are PCR tests for COVID-19?
New research calls into question the accuracy of SARS-CoV-2 RT-PCR tests as the chance of receiving a false negative COVID-19 test could be greater than 1 in 5.
During the current rush to perform and publish research to tackle the pandemic, some efforts have been made to help prevent that rush from translating into mistakes. For example, one web resource contains validated SARS-CoV-2-related structural models from the Protein Data Bank [5]. The resource explains that its creation was due to concern over the ‘accelerated mode’ of this research and the elevated chance of mistakes that it brings.
This effort is commendable, yet it relies on the data from the Protein Data Bank being openly available for validation. Many data sets are often unavailable – especially in clinical research, where de-identification can be tricky [6] and data are often only made available upon reasonable request after publication of the study, through an email to the corresponding author. What’s more, with increased throughput, complexity and interdisciplinarity within research, more companies – such as Surgisphere – are cropping up to support scientific research (see [7] for an example of the sheer number). This could lead to the gatekeeping of data owing to the protection of intellectual property, and the data aggregators being separate from the research team.
These issues are supported by a lack of standardization in how data is archived, reported, published and cited [8]. While this has improved in recent years, the recent retractions highlight that problems remain.
All in all, these retractions highlight that while steps have been taken, we still have a way to go to ensure the data behind studies can be relied upon. I suspect this will need to be driven by all parties – researchers, data aggregators and reviewers need to ensure data are compiled and analyzed appropriately, funders and stakeholders need to support and mandate standardized and open data policies, and publishers need to ensure data policies are adhered to, and peer review is appropriate.