Integrating microbiomes into clinical trials – the importance of time
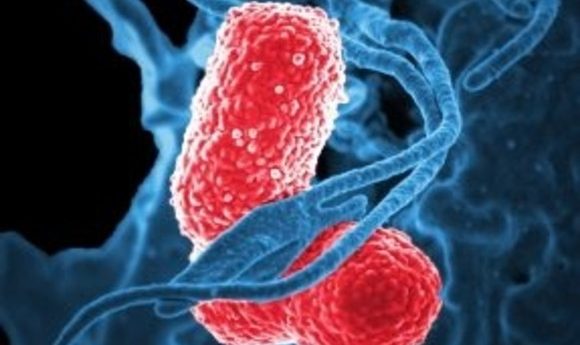
Jack Gilbert (University of California, San Diego; CA, USA) and Sean Gibbons (Institute for Systems Biology; WA, USA) explore the challenges faced when incorporating microbiome and metabolome data into clinical trial design, due to the inherent longitudinal variability in the data, and propose a solution to help overcome these issues.
The integration of microbiome and the concordant metabolome data into clinical trial design has enormous value for a range of diseases. However, it is still unclear how exactly this integration should be achieved. While the multi-day average composition and structure of an individual’s microbiome and associated metabolome is quite stable (i.e. barring major shifts in diet, health, and lifestyle), the relative proportions of microbial and chemical species can show substantial variability on day-to-day timescales [1]. Short-term variability in microbial and metabolic time series introduces noise into cross-sectional analyses, which in turn reduces the likelihood of identifying useful clinical biomarkers of disease and substantially increases the rate of false positives. Additionally, monitoring this short-term volatility could be valuable for identifying important mechanistic insights into variable responses to treatment (e.g. responders and non-responders) across a population.
The proliferation of clinical studies that include microbiome and metabolome data has been predicated on the potential of these efforts to identify reliable diagnostics and improved therapeutics. However, there have been few major successes so far. This may be due, in part, to flaws in the study designs, which often do not take into account the day-to-day noisiness of multiomic measurements. This ‘research noise’ is quite common in the early development of a field, but as the promise of microbiome and metabolome investigations becomes more evident and the field matures further, the need to elucidate the optimal experimental design for capturing quantitative associations between microbial ecology, biochemistry and health becomes vital. It is important to set ourselves up for success and show that microbiome science can be successfully translated into the clinic.
The current tools we use to investigate microbiomes or metabolomes have many biases and limitations, which preclude their universal application across diverse clinical trial designs. The most obvious flaw is that measuring the microbiome or metabolome is not like measuring blood pressure, in that it is not the quantification of a single, isolated variable. Rather, untargeted omics data are complex, compositional measurements of the relative frequencies of hundreds or thousands of features that are extremely sensitive to the conditions under which they are generated. These data require specialized statistical models and computational tools that can take compositionality, sparsity, batch effects, technical noise, sampling noise and spatiotemporal variation into account.
While many of these tools now exist, developers are still hard at work designing new-and-improved analytical frameworks, especially to account for a rapid increase in data density and complexity, which increase computational time and cost. In addition to analytical tools, the collection of supplemental data – like qPCR, flow cytometry or spatial context using microscopy – can also improve multiomic analyses. For example, longitudinal sampling of an environment (i.e. oral saliva, stool, skin, vaginal mucous, etc.) can yield more accurate estimates of steady-state feature frequencies, which in turn can improve statistical inferences. However, repeated sampling is a heavy burden on patients. Standard approaches require the patient to sample themselves directly, either having to swab or wipe specific areas or sample their own feces, which is often considered unappealing and can lead to other problems with shipping and storage of samples. Having to repeat this process over multiple days, or ideally at even higher temporal frequencies for prolonged durations, is unlikely to be viable under the current paradigm.
New approaches are needed to reduce the burden of longitudinal sampling for patients. The development of technologies to enable automated sampling of fecal matter integrated into a participant’s standard toilet regimen (e.g. [2]) will alleviate the burden for stool analysis, but further work is needed to enable similar technologies for other body sites. However, the optimal number of longitudinal samples and the best temporal frequency for improving statistical inferences still remains to be definitively quantified.
Fortunately, recent data can shed light on timescales of variation in the human gut microbiome. In the absence of major perturbations (e.g., antibiotics, food poisoning, etc.), dominant bacterial taxa in the guts of healthy people maintain fairly consistent average abundance levels over months-to-years. Day-to-day fluctuations can deviate substantially from this average, but recovery back to the steady-state abundance tends to be rapid (i.e., autocorrelation decays within a matter of 3–5 days). Recent timeseries analyses have shown that between 5–9 time points, spaced 3–5 days apart, are optimal for estimating the average abundance level of a given bacterial taxon in the human gut [3]. Collecting fewer time points results in a noisier estimate of this average while collecting more than nine time points doesn’t appear to improve estimates any further. Collecting three time points per person would be sufficient to markedly improve within-person mean frequency estimates. However, this estimate only accounts for healthy individuals; participants who are immunosuppressed or on a microbiocidal drug therapy may show substantially different dynamics.
Additionally, different clinical trial strategies will require heterogeneous observation periods, which should be tailored to the needs of the trial. For example, if exploring the association between microbiome-mediated inflammation and side effects or recovery for a chemotherapy drug, long durations (e.g. >30 days) of continuous measurement may be required to account for potential heteroskedasticity within a disturbed gut ecosystem during recovery and alternative kinds of dynamics across the population.
Here, we present a basic rule of thumb and suggest that simple cross-sectional clinical trials should integrate a minimum of three and a maximum of nine ‘omics data time points per patient (each time point sampled >2 days apart) in order to improve signal-to-noise and increase statistical power. This simple fix could save time and money in the long run and accelerate the translation of microbiome science into clinical practice. However, as few long-dense time series exist for unhealthy patients, we do not yet know whether the optimal number of time points varies across clinical populations. The variability in clinical trial design elements (e.g., cross-over trials, wash-out periods and acute vs chronic outcome measures) and the probability of uneven sampling frequencies (e.g., during diarrhea or constipation episodes) also need to be taken into consideration. Future work should focus on identifying these optima by producing long, dense time series (i.e., >30 daily samples) in many different clinical trial scenarios. By accounting for within-patient temporal variance in multiomic data, we can improve our statistical inferences, identify promising biomarkers and therapeutics, avoid false positives and increase the overall efficiency of clinical trials.