Nothing’s perfect, but predicting Alzheimer’s onset nearly is
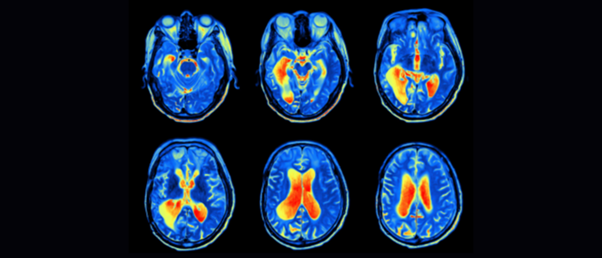
Deep learning–based prediction of Alzheimer’s onset from MRI scans boasts 99% accuracy.
Kaunas University of Technology and Vytautas Magnus University (both Kaunas, Lithuania) researchers have developed a deep learning-based method that can predict the possible onset of Alzheimer’s from MRI brain images with over 99% accuracy. This provides a highly accurate and time-efficient method for professionals to analyze fMRI images for these signs to improve correct diagnosis and provide treatment at an early stage.
“Medical professionals all over the world attempt to raise awareness of an early Alzheimer’s diagnosis, which provides the affected with a better chance of benefiting from treatment,” commented author Rytis Maskeliūnas (Kaunas University of Technology).
Mild cognitive impairment (MCI) is a stage between the expected cognitive decline experienced with typical aging and that of dementia. Early MCI stages often have almost no clear symptoms; however, a useful indicator of signs of potential early onset of AD can be detected using fMRI scans of the brain.
Current manual analysis of fMRI images to identify predictive AD-associated changes requires specialist knowledge and is heavily time-consuming. Therefore Maskeliūnas’ team aimed to utilize a deep-learning model for the analysis of fMRI images in order to provide an effective and time-efficient solution.
“Modern signal processing allows delegating the image processing to the machine, which can complete it faster and accurately enough. Of course, we don’t dare to suggest that a medical professional should ever rely on any algorithm one hundred percent. Think of a machine as a robot capable of doing the most tedious task of sorting the data and searching for features. In this scenario, after the computer algorithm selects potentially affected cases, the specialist can look into them more closely, and at the end, everybody benefits as the diagnosis and the treatment reaches the patient much faster,” described Maskeliūnas.
Regular exercise could help prevent onset of Alzheimer’s disease
Regular physical exercise has been shown to help modulate iron metabolism in the brain and in muscles in a new study.
Maskeliūnas’ team developed the deep learning-based model using a modification of ResNet 18 (residual neural network) to categorize 138 subjects’ fMRI images. The images were grouped into six different categories: normal control, MCI, early MCI, late MCI, significant memory concern, and AD. For training and validation of the model, 51,443 and 27,310 fMRI images were selected respectively from The Alzheimer’s Disease Neuroimaging Initiative dataset.
The model was able to effectively find MCI features in the given dataset, achieving the best classification accuracy of 99.99%, 99.95% and 99.95% for early MCI vs. AD, late MCI vs. AD, and MCI vs. early MCI, respectively.
“Although this was not the first attempt to diagnose the early onset of Alzheimer’s from similar data, our main breakthrough is the accuracy of the algorithm. Obviously, such high numbers are not indicators of true real-life performance, but we’re working with medical institutions to get more data,” added Maskeliūnas.
Researchers postulate that the model could be developed into a more comprehensive software, which would analyze data from groups more vulnerable to the development of AD, such as the over 65s, those with a history of brain injury, and high blood pressure.
Further down the line, the model could be combined with additional systems to analyze multiple parameters, such as eye-movement tracking, face reading and voice analysis to improve the predictive power. The future prospect of this model could allow for self-checking services and flagging patients and medical professionals to early warning signs of Alzheimer’s development.