Fast-tracking the future of genetic testing
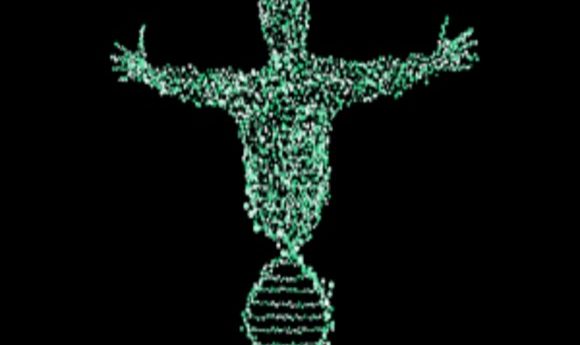
Carlos Araya discusses a new direction for clinical genetics and mutation analysis as well as how this can be used for therapeutic development.
Carlos Araya gained a PhD in genome sciences from the University of Washington (WA, USA) before moving to Stanford University (CA, USA) to carry out his postdoctoral research in genomics and biophysics. It was here that he co-founded Jungla (CA, USA), an AI-driven Biotechnology company that aims to give robust and scalable clinical guidance in the field of genomics and genetic testing.
Please could you give us a short overview of why you started Jungla and its main aims?
Jungla was started by three co-founders from Stanford University, all from technical backgrounds though different areas. We came together with a shared perspective and vision: that the ability to sequence and record genetic data has become much easier and cheaper but our ability to use this information hasn’t advanced at the same pace. This means that though there is a lot more information available to us, the fraction of this that we can use is still quite small and so our ability to understand what those mutations mean is relatively limited.
The reason for this is largely because we have a clinical genetics and genomics testing framework that is largely observational, meaning that for each piece of genetic information we have to see enough individuals with or without a condition to allow us to determine a genetic association. This allows us to determine associations for mutations that are common in the population though it makes it difficult to understand the ones with a low frequency. The majority of mutations are rare, often found in less than 0.01% of the population or even private to families or individuals.
Therefore, the current observational frameworks where you’re required to have seen enough patients with a disease or enough healthy individuals just can’t provide enough information. This results in clinical tests that are often unable to give a diagnosis as, although a mutation is found, it is unknown what these genetic alterations mean. Even when a known mutation is found, it is likely that the individual will have other confounding mutations.
Following this vision, we formed Jungla with the overarching goal of moving the clinical genetics and genomics framework from an observational to a model-driven approach. One of the main reasons for that is so that we can make quantitative models that have the ability to give metrics for each interpretation that is made and suggest the likelihood that mutations are disease causing or not.
These original models will allows us to build a network of labs, working together to improve the quality of the model and support each other so it creates a collaborative intelligence infrastructure. As we integrate modeling into clinical practice, we can incorporate data from each individual, allowing the information from each patient to help improve the care of the next rather than simply others with the same mutation.
How are you currently utilizing artificial intelligence (AI) technology in your models to improve clinical genetic tests?
We use AI in a lot of ways, at many different stages in the process and in many different forms.
The current observational approach has given us information for mutations that we know vary in representation in affected individuals versus unaffected individuals, giving a picture of some number of mutations that we can provide answers for today. We first access that information and quality control it with AI to make sure that there is a well curated definition of which mutations definitely do and which definitely don’t cause disease, and determine confidence metrics for each.
From this data we can then use biological and additional AI tools to predict all of the other possible mutations that can happen in each gene and determine those important for each disease; we need to be able to answer the question of whether a mutation is disease causing or not in essentially every gene. We don’t know which mutations we will need to provide answers for first as we don’t know which patients will show up to the clinic first; as a consequence, we need to proactively generate answers for all of them and do so quantitatively.
To try and find these answers we use AI to take that body of knowledge that exists today, refine it and use it to project out what the likelihood of causing disease is for all other possible mutations.
Could you explain a little about the technology platform that you have created?
Jungla has developed a Molecular Evidence Platform (MEP) which continually surveys knowledge regarding the clinical significance of genomic variation in patients, integrates this knowledge with proprietary molecular and cellular data, and a wide repertoire of AI techniques, to ultimately generate, validate, audit and then distribute high-quality clinical guidance to the industry.
With the number of genes routinely tested in the clinic and the large numbers of mutations possible in each gene, there are millions of possible mutations we need the answers for, of which we only know a small fraction. The MEP provides information for all of these potentially disease causing mutations for each gene by continuously updating and quality controlling the knowledge gained from clinical testing across hundreds of thousands of patients. The MEP can then generate computational and cellular models that measure how these mutations affect the biology of either individual molecules or cells as a whole. The results from these models allow it to predict which mutations are disease causing and which are not by figuring out which properties of the molecules and cells are important in each disease.
The way we at Jungla see it is that these molecules we are modelling are biological elements with a really wide diversity, each with very complicated behaviors that are not described by just their sequence in the genome. These are living, physical machines that are doing a lot of work both individually and in complex networks, and which mutations can affect the functioning of in many different ways.
As the MEP learns to make predictions of the clinical significance of mutations, it derives measures of confidence for each one so not only can it make these predictions but it can also give metrics as to how confident the MEP is, ranging from high confidence to low confidence. This allows us to tune the performance of the MEP by controlling which predictions we distribute to partners. If only looking at 80% of the mutations gives a higher accuracy than looking at 100%, then we can restrict the MEP to providing only the most reliable answers.
To ensure transparency and a basis for trust, we’ve also made it so that every time we create a model the predictions and scores of confidence are stored in a blockchain which allows us to show that we made specific predictions at specific points in time. This means that as knowledge for mutations is learned through the standard practices in clinical testing, we can continuously measure how accurately the MEP predicts the results of future clinical tests in each gene and disease. Essentially, this allows us to be always looking at how well Jungla is predicting the state of clinical knowledge as it emerges. Genetics is an evolving picture and with this system we can assess how well we are predicting this developing body knowledge. We’ve been able to demonstrate that the MEP provides very high accuracy for millions of mutations, across thousands of genes.
Could you talk about the new technology platform you have created and your collaboration with Hewlett Packard (HP)?
Jungla is developing and investing seriously in state-of-the-art models that are increasingly mechanistic, both computational and cellular.
The work with HP is an example of this, where we are extending our use of biophysical simulations to assess how mutations can change the behavior of molecules and how these changes in behavior explain the pathogenicity of variants. Using memory-driven compute infrastructure, where we can for example load into memory 4,000-times the amount of data that conventional computers can load, we can perform very high resolution analyses and obtain mechanistic insights into the how and why these mutations work. Whereas there are a plethora of companies touting genomics and AI, Jungla delves deep into molecular and cellular mechanisms. As you move from the basic sequence information to detailed molecular and cellular data you get a large explosion in the volume of the data; while the sequence data from an entire genome can be stored in gigabytes, the detailed data we are using can take up to 40 terabytes just for one gene and its clinically-understood mutations.
Working with HP we are showing that we can use these methods that, though established in the biophysical field, are not used in genomics. As a genomicist, I can say the genomics field is great at generating and characterizing variation in sequences at a high level, but truly understanding how molecules and cells function is beyond its purview. As a consequence, the clinical genetics and genomics communities –we believe– have been underpowered, unable to integrate and leverage advances from adjacent fields.
Analyses requiring comparisons against 40 terabytes of data come with a large series of computational challenges so using HP’s large memory systems has been really powerful; it allows significant acceleration in the speed with which this data can be analyzed. This has allowed us to analyze and characterize the data roughly 200- to 250-times faster than it would take to do on conventional infrastructure. This is critical in a clinical setting where waiting 250 days for an answer is not viable. What this allows us to do is apply the most sophisticated approaches from science at timescales that are clinically meaningful. This is what it takes if we are to bring state-of-the-art tools from science and engineering to serve patients.
What do you hope for the future? Where do you see this technology going in the next 5-10 years?
Currently there seems to be this idea that these questions are all going to be answered by just sequencing more patients; the thinking (as I understand it) is that if we keep getting more observations eventually we will have enough to cover everything. We fundamentally disagree with this view, and believe that even if it were to be proven true, we will be waiting a long time until we’ve not only made the measurements but also statistically sampled all of the possible correlations between the mutations and the disease. In addition, this observational approach will simply not explain the mechanisms by mutations cause disease and hence will never allow us to translate these observations into improved treatments.
Our view is that, instead of waiting for all of these observational measurements to be made and shared, we can models generation, quality control and distribution to the industry thus creating a form of collaborative intelligence. If we bring modeling to directly support individual institutions in their efforts to provide accurate and efficient results to patients, then each one can provide improved clinical tests to their patient populations while contributing to improve the same for others. As a whole, everyone – most importantly patients– benefits.
As these models become more mechanistic there’s an opportunity to use them to understand how all of these mutations are affecting our biology to cause disease. If we can understand these mechanisms, we should then be able to apply the knowledge to inform the design and application of interventions that could help to ameliorate the symptoms or the condition completely. If we develop a quantitative and mechanistic understanding, then not only can we improve our diagnostic ability but also use it to improve and speed-up therapeutic development.
That really summarizes the future goals of Jungla; one, to make the genomics-based diagnostics quantitative and model-driven, and two, to create and translate mechanistic insights from clinical genomics into better therapeutics.