AI: directing drug discovery towards success
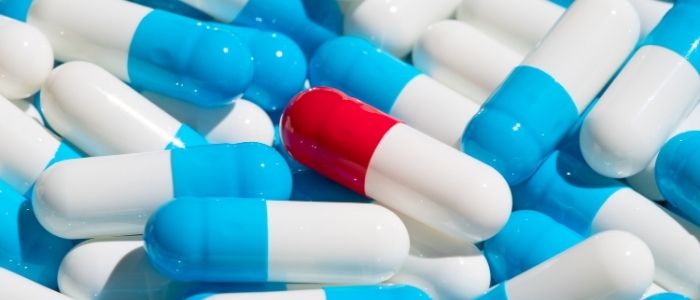
Drug discovery is a lengthy, expensive and perilous process. With only 14% of the drugs that make it to clinical trial stages finding success, the process needs an upheaval. Could AI and a shift in perspective from a singular, target-driven approach to a broader, phenotypic approach help improve the process?
Peyman Gifani is the CEO and Founder of AI VIVO (Cambridge, UK). His background is in biomedical engineering and he took his PhD at Cambridge University. Peyman’s major interests during his academic career have been in control engineering, machine learning and systems biology, which has led to an interest in using engineering concepts to analyze biological systems. It is this interest that is at the core of AI VIVO.
Why do you believe the current drug discovery process has such a low rate of success?
The current drug discovery process is dominated by a target-oriented approach, one based on a simplistic view of treating a disease by modulating one target gene using one drug. Such linear thinking rests on plausible logical linear causation that might work only for certain monogenic diseases, like a cancer driven by a single dominant oncogene. However, biological systems consist of millions of components (genes, proteins, metabolites) forming interconnected networks that influence each other in a nonlinear manor, exhibiting a collective, emergent, dynamic behavior.
In the field of big data medicine, there have been huge efforts to capture the architecture of such complex networks using AI-based text mining to extract information from the literature and to build large scale graphs that represent the connections in such networks. However, such network architecture is still essentially a static entity and is viewed as wiring diagrams or maps. Here is where the explanatory utility of such approaches based on static network architecture ends. This explains why Pharma has been plagued with repeated failures in recent decades.
What is your company’s proposed solution to these issues?
AI VIVO provides a systems pharmacology platform based on a phenotypic approach to the drug discovery process. Inspired by dynamical systems theory from physics, AI VIVO considers network dynamics as the key concept for linking network architecture and cell behavior. This approach explains both the cause and effect of changes in cell. AI VIVO’s approach is far more than listing correlations and inferring causation from literature or omics databases. This enables AI VIVO to lay out a logical path to answer a biological question driven by a hypothesis rooted in a dynamical systems theory and in rigorous medical knowledge.
This platform involves a mathematical model that uses functional genomics data to represent the effect of diseases at the phenotypic level and at different stages of disease progression. We call this the disease manifold. This is coupled with a similar model that represents the cellular effects of different drugs – the drug manifold. This recognizes the fact that human disease is dynamic and not static, every disease state has a unique manifold. Similarly, each compound subsequently used to treat disease has a manifold depicting how it changes the state of a disease.
We currently have information for more than 500 different disease areas in total, with over 800,000 different manifolds for different disease stages.
How does AI VIVO use these manifolds to identify and rank promising drug candidates
In short, the manifolds enable AI VIVO to search various disease stages and identify corresponding targets, mechanisms, pathways, indications, and compounds, which can lead to effective and often unexpected treatment strategies.
The manifolds are used by our prediction engine in a disease-oriented approach to highlight which cellular systems are affected by a disease and require modulation, matching this requirement to compounds or a combination of compounds that can produce that kind of modulation. These potential compound combinations are then ranked by the prediction engine for their chance of success.
After this rank is established, we can then do some quality control, using additional criteria and experimental validation to assess the compounds and further judge the likelihood of success for the drug. We do this by asking a few questions: Why is the drug highly ranked? Is it logical or surprising that it is highly ranked? What do we know about the drug at the moment? This examination is conducted by biologists and disease experts.
If you are studying a well-known disease area, you would expect to see some established drugs for that disease at the top of the list, indicating that the ranking system is working well. You can then look at the unexpected or unexplored drugs at the top of the list so see if they could have a greater impact.
This approach allows you to consider all potential drug targets for a disease and can yield unexpected links between diseases and therapeutic compounds, leading to new ideas for drug repositioning and drug combinations.
There is also a second drug-oriented approach, which is essentially the reverse. We start with a drug and create a manifold for it and then look to see what additional diseases that drug could be used to treat. The asset can be preclinical or clinical compound candidate. This will lead to indication discovery & drug repositioning.
AI is here to supercharge pathologists, not replace them
AI and automation have started to replace jobs in some industries. However, healthcare, particular pathology, should not be in this group
How has this platform been used to combat COVID-19?
In February 2020, we asked ourselves the question: “can we build a disease manifold representing COVID-19?” We spent about two weeks collecting and generating the required functional genomics data that we needed to answer that question.
We then started by building a phenotypic model of the impact of COVID-19 on the lungs using real samples from COVID-19 infected cells – and then used our platform to identify unexpected compounds that may treat COVID-19 by moderating the undesirable phenotypic changes that it causes.
We used our prediction engine to rank top drug candidates, assessing around 90,000 different manifolds. We then focussed on the top 0.3% of the total ranked candidates, filtering for approved and generic drugs plus some candidates from Phase II and III clinical trials.
What was interesting is that our disease model had highlighted the hyperinflammation and overstimulation of the immune system – a cytokine storm – as a key aspect of the disease progression.
The results have far exceeded our expectations. In April we were able to release a list of candidates into the public domain, which included dexamethasone. The low-dose steroid treatment, has been part of the world’s biggest trial testing existing therapeutics that could be repurposed to help treat COVID-19. The drug, which is inexpensive and widely available, was shown to save the lives of seriously ill patients at a late stage of coronavirus 10 weeks after our discovery. This was viewed globally as a major breakthrough in the fight against the deadly virus. We have updated and published this list and as of a few days ago, 48 of our top ranked list candidates are in clinical trials for COVID-19.
What impact do you predict that AI VIVO will have in the next 5 years?
On the COVID-19 side of things, we have been in contact with different clinical trial investigators around the world to help them find better drug combinations. We are very pleased with the success of dexamethasone but we believe it is only addressing one aspect of the disease, so we have used our platform to rank further drug combinations.
I believe AI itself is not the main solution for the problems we are facing in pharmaceutical industry.
In drug discovery in general, I believe we need to change the whole model, to transition from the dominant target-oriented approach to a broader phenotypic approach that takes into account multiple targets at once.
Machine learning based approaches still follow the traditional linear development strategy in which the only reliable experimental models are at the end of the pipe. We need better predictive in-silico models, which is what we are trying to address using our manifolds technology.
We still don’t understand how life works at a molecular level and our interpretative frameworks are flawed. We believe the AI-based text mining and knowledge graph techniques are not reliable as in many cases the sources of information (e.g., scientific papers) are not reliable and reproducible. We ignore this background information and only uses functional genomics data as an initial step to remove bias from the AI VIVO ranking system. Using functional genomics data enables us to purely present the disease phenotypically and improve the chance of finding multiple unexpected ways for disease modulations. This will also enable us to predict innovative combination therapies. Finding drug combinations remains one of the biggest challenges for the industry at present.
We believe our disruptive technology enables us to speed up the whole discovery process by identifying multiple promising unexpected candidates concurrently, reducing the risk of pursuing various opportunities through preclinical validations, and progressing drug candidates to the clinic through industry and investor partnerships.