Integrating artificial intelligence and genomics
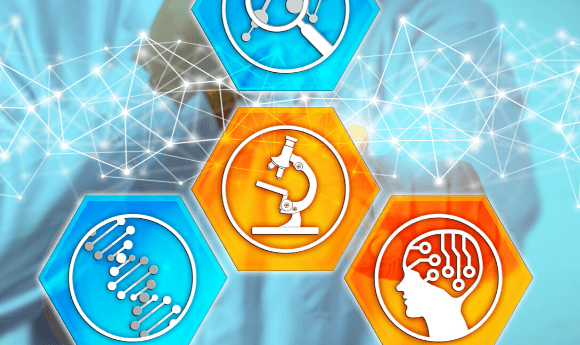
As advances in sequencing mean access to genetic data becomes easier and cheaper, the volume available to analyze is growing beyond the scope of human capabilities and the need to incorporate artificial intelligence (AI) into this analysis is becoming more apparent.
BioTechniques caught up with Emedgene (CA, USA) Co-founder, Niv Mizrahi, and Director of Marketing, Orit Livnat-Levi at ASHG 2019 to discuss how Emedgene is working to overcome the challenge of increased data volume.
About the Team
Niv Mizrahi: Niv is the Co-founder and CTO at Emedgene. Having gained degrees in both physics and computer science from Tel Aviv University (Israel), he is an expert in big data, machine learning and large-scale distributed systems. In his previous role as Engineering Director at Taykey (NY, USA) he has gained experience in leading developers to successfully implement multiple complex big data systems.
Orit Livnat-Levi: Orit is the Director of Marketing at Emedgene. Predominantly working with startups, she has worked as a consultant and helped to grow the marketing departments of new companies for many years. She left her consulting job to join Emedgene at the beginning of 2019.
In your opinion, what are the current challenges in genetic data management?
Niv Mizrahi: We always say that sequencing is easy, interpretation is hard – especially as the cost of sequencing is decreasing. About 4 months ago the Illumina (CA, USA) CEO said that they were going to push their numbers to $100 by 2022. We will have to see if this will occur in their timeframe but the whole industry is waiting to see a $200 or $100 genome. However, even as the price of sequencing decreases, people still spend a lot of time going through reports, going through variants and reading through articles in order to actually come up with results. Then, most of the time they don’t have any results relevant for patients, so they have to come back to those cases again and again, 6 months later, a year later, a few years later in order to solve it. This shows that interpreting results is actually the main labor and is probably the hardest thing in this industry – that’s where we come in to play. We try to build machine learning and AI toolkits that will each solve parts of that problem until you have a single flow that is an end-to-end, automated solution.
Orit Livnat-Levi: Let me just add that those geneticists interpreting the variants, there are a few thousand of them in the world so there is no way we can grow genomics-based medicine with human labor alone.
Niv Mizrahi: I think the main difference is, for example, if you look at microarrays, there isn’t that issue because all the variants that you are looking at are already known. However, once you look at next-generation sequencing, each person that you interact with has private variants that no one else in the world has, even non-articles. You have to be able to find a way to interpret it and look at similar variants and still be able to deduce a clinical decision from all the other information that you have on that variant. In Israel, we are lucky because we have a lot of labs that are working with us and collaborating. In places where labs are not sharing the right information and the process is not automated, you can end up in situations where, for example, one lab finds a variant in a fetus and recommends an abortion as a result of that finding while another lab will see it as benign. This highlights that having a standard decision in this field is really important and there is a lot of work there; the ACMG guidelines is one and the ClinGen work from the NIH (MD, USA) is the second one, but to be able to do that in scale and not only in a few dozen genes – that’s the main challenge.
What is Emedgene doing for this field?
Niv Mizrahi: Emedgene provides an automatic interpretation platform. We currently have two main products. One is Wells; Wells is a genomic interpretation workbench that allows geneticists to go through cases and to look at phenotype information they have gathered, to go through the family history and to store all the different genetic information that is involved in each case. Then they actually have the workbench platform and can tag different variants, communicate within their team and build any necessary reports. There is a really robust filtering system meaning researchers can add their own standard operating procedure (SOP) into the platform, so if they work in a certain standard of operation and each time they are acting out specific steps in order to go over a case, they can actually build those filters into the platform and make sure every person goes through those steps. They can create whatever pipeline that they want and it is really customizable in a sense that they can build their own workflow, like having Sanger sequencing or not and so on.
Orit Livnat-Levi: Let me just say on that solution, it’s a really, really good tool in what is quite a competitive market because a third of the Emedgene R&D team are geneticists so they are influencing the user interface decision, the flow decision, every decision in the platform. This means that when a user looks at it, they should be able to say “oh it’s exactly like my workflow and it’s so intuitive”. We have had people in demos just grab their keyboard and mouse because it’s so intuitive they don’t even need training on it.
Niv Mizrahi: The second product we have is called Ada and I think it is where we shine as a company and all of our data scientists and geneticists start come in to play. It’s a set of algorithms that play together in order to automate this process in terms of finding the genes that are causing diseases for a patient phenotype. Our natural language processing engine goes through articles, builds facts out of them, aggregates these facts and predicts new gene—disease connections and other connections through mouse models, pathways and secondary connections. We have identified close to 1500 unique genes that are known to cause diseases and are currently not known in any public structural database.
- Big data in big trouble?
- Innovative smart device could increase laboratory productivity
- Artificial intelligence to predict cancer immunotherapy response
How does this platform outperform existing datasets?
Orit Livnat-Levi: So, if you think of the big databases that everybody relies on, they are manually curated which takes a long time. We identified a gene back in 2016, and it’s still not available in any database because it just takes that long.
Niv Mizrahi: It is the first case that I solved using the platform on my own. I do not come from a genetic background, so it comes to show how intuitive the platform can be. That’s one of our strengths in terms of utilizing articles, we identified a strong publication on that gene, which included a convincing knockout model, and since the algorithm surfaces candidates based the literature, not just databases, it suggested this candidate.
Orit Livnat-Levi: Many of the players in this field that have some sort of prioritization algorithm though they would usually integrate it after the interpretation process. However, our algorithm has gone through a rigorous validation study with Baylor Genetics (TX, USA), and achieves outstanding results. Our algorithm is the only one at present that can factor in connections from the literature, which reduces time spent per case and increases the likelihood of identifying variants that have been covered in the literature and not in databases.
What would you say the most important algorithm is in Ada?
Orit Livnat-Levi: Well the most important one is that we pinpoint the pathogen variant. We present ten variants instead of the 500 that you might see in a typical pipeline. For those ten we ran a valuation study with Baylor Genetics in a cohort of 180 cases – 96% of their time we found the causative variant in the top 10. It’s 98% in the top 20 so really strong results. It is worth noting how similar the study cohort is to an actual production cohort; we did not play around with anything. Those are 180 randomly chosen Baylor cases with a breakdown of specialties covering all that they analyze. That is probably the main algorithm but then when we pinpoint one specific variant we are able to then display evidence.
Niv Mizrahi: Someone could potentially build a perfect algorithm; it will show only one variant and it will be the one, but it might still not be that helpful for a geneticist. They would still have to dig through the articles and search for the right context that they need to see. The idea of our algorithm is that the type of information for each specific variant, which the geneticist needs to look at in order to make the decision the fastest, is displayed to them. We actually show the different connections, the different articles and data points that they need to see in order to deduct the different steps and to make their final decision that the variant is causing the patient’s disease. It can show how the variant is connected to the gene, if there are similar variants or if it is found in an article. Then, as well as showing that connection it is also explaining it. If it says a variant is in a given article it can also state what its presence in the article may indicate and it can deduce lots of other information.
All this information can then be displayed in a concise way for the variant interpretation scientist to read off a visual information sheet. We have a few different ways to visualize the data, because different people consume data in a different way. One way is to use a graph that shows the connection between variant and article with links between them and an explanation on each link that displays the actual resources that made the algorithm deduce this connection. A lot of people really like it; I find that personally it really helps me to make a decision, but some don’t consume data visually and they have to read. Therefore, we also have a textual representation, just like an abstract summary of an article, which explains all of the different connections and allows people to just read it. Different ways work for different people, but the main idea is to highlight which data points are necessary for the geneticist to view and understand in order to deduce the same conclusion as the algorithm.
What is next for Emedgene?
Niv Mizrahi: Our latest algorithm is called Pathorolo, which means solved or not solved in Hebrew. It’s an algorithm that can actually predict whether a case is solvable for a geneticist. There are a lot of different uses for it; the first one, or the easiest one, is the reanalysis flow. Labs are continuously getting more and more cases but often only solve 25–40% of them; it depends on how good the lab is, which means 60% of their patients are not getting anything back from these tests.
What a lot of labs are currently doing is just going over every single case, despite it potentially being unsolvable, which is unsustainable in the long run. If they are in max throughput, which means it took them a year to do 100, they can’t do a further 60% with the same amount of time needed to invest in the analysis – it just doesn’t make sense. The idea of this algorithm is that we can pinpoint in a list of past cases which ones are solvable with the new information that is currently available today. The knowledge gap is constantly updating with new literature and all the different variant databases and data points available out there are also updating, so now we can identify which of the old cases have up to a 90% chance of being solved now or which remain unsolvable. This means that you can be sure you are investing any time you put into past cases into the right ones. That’s one utilization for this algorithm and we have great results there and the lab can decide on the threshold they are looking at.
Orit Livnat-Levi: The algorithm gives every case a score, so all cases that score 0.75 and higher have a 91% probability of being solved, which is really positive so you will want to revisit those. On the flipside, if cases have a really low score like 0.2 then there is a 90% chance that it won’t be solved
Niv Mizrahi: This is another use for the same algorithm, just looking at the data from the other way around. Most labs have their SOP that they are going to follow when they are looking at a given variant and they have things they need to check for. In the platform, they can create their SOPs and check in a checkbox when they have done each step. They can always dig deeper and spend more time on it if they wish though if they solve the case and identify the variant, they don’t feel the need to dig further. However, when they don’t solve it, some geneticists will feel their urgency to keep digging and try to solve it even though it may be unsolvable. If Pathorolo says you have a probability of less than 25% of solving the case you know that no matter how much you dig you are unlikely to solve it. In the validation set that we went through, 90% of the cases with a Pathorolo probability of less than 25% were not solved in the end.
Orit Livnat-Levi: It helps to give an indication of the outcome. Still follow your SOP, it is an algorithm and you shouldn’t base a decision on the algorithm alone, but it does let you know that you don’t have to dig it to infinity. It’s highly likely that the information just doesn’t exist yet, we don’t know enough to solve that particular case.
Niv Mizrahi: It is just a way to say, okay, don’t spend too much time on that case right now. We think it will be really effective for the research environment because a given group might not have an SOP or they just have a huge backlog of cases. If said group is only a small team of five or ten people but has upwards of 500 cases to go through, they just don’t know where to look first. This algorithm can help them to prioritize their queue and go over the ones that are solvable first because it’s helpful to just get them crossed off your list before you dig deep into other cases.
We think we will see a lot of different utilizations for this algorithm and it has already had preliminary validation in a study with Baylor Genetics, though we are hoping to perform further studies with other partners in order to validate it in other labs.