The data of a pandemic: how has data influenced our understanding of COVID-19?
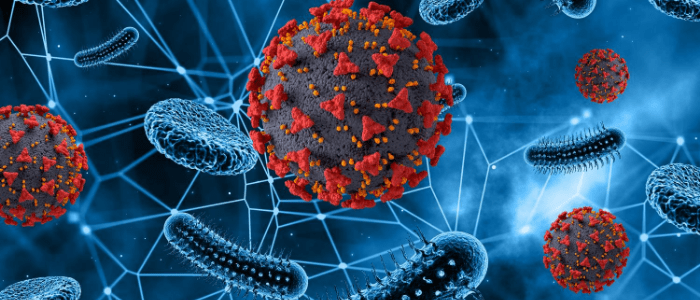
From predictive models to contact tracing, data has been key to understanding the COVID-19 pandemic.
As the COVID-19 pandemic progresses, the amount of data available has increased exponentially. Be it information on new cases, potential drug candidates or personal information for contact tracing, the volume of information that is required to be processed is vast. To cope with this influx of information, artificial intelligence and machine learning algorithms have been applied like never before in order to improve understanding of SARS-CoV-2, track the spread of COVID-19 and, ultimately, contain the outbreak. Real-time disease trackers, as well as models predicting future developments, have been used by governments worldwide to guide policy decisions and prepare healthcare professionals.
Data has been key to driving our understanding of SARS-CoV-2 and the disease it causes. Here, we explore some of the data-driven techniques that have been used, as well as highlight some of the shortcomings and gaps in the knowledge.
COVID-19 data-driven predictions
On 23 March 2020, the UK Government (London, UK) changed their coronavirus procedure from one based on developing herd immunity to one imposing a strict lockdown, effective immediately. The U-turn in protocol was due, in part, to an epidemiological model developed by researchers at Imperial College London (UK) that predicted that, should the UK continue on their original trajectory, it could result in upwards of 250,000 deaths. Across the globe, data-driven infectious disease surveillance and prediction modeling became key for determining government policy, and models were created for different countries, scenarios and policies utilizing all data available.
Publicly available data sets, such as this one maintained by Our World in Data, have allowed statisticians and epidemiology experts to develop extensive models and predictions, each one running under a different assumption of the virus to predict a different outcome.
Modeling Covid-19 is one such model, created to play out different social distancing scenarios across various locations. Starting by predicting the spread of the virus were social interaction uninterrupted, the team is then able to identify the value of social distancing and find out the optimum compliance needed to have a significant effect. The model applies the commonly used SEIR model, which estimates the future spread of the virus by splitting the population into six groups: Susceptible people, exposed people, infections people, hospitalized people, recovered people (assuming a long-term protective immune response) and deceased people.
Building on the classical SEIR model, COVID-19 Projections incorporated machine learning techniques in order to minimize the error between their projected outcomes and the actual results. The machine learning algorithm is able to determine the best values for the variable parameters of the model, such as R0, mortality rate etc., based on real-time data from the real world. This adjusts the projections so that they are the best fit for the future based on the current situation.
Of all of the models available, none will be 100% accurate at predicting the future. They are also likely to disagree, as they are each using a different type of mathematics and modeling a slightly different scenario. Looking at multiple models and understanding how they differ is likely to give a more comprehensive view of the direction the disease is heading, as demonstrated in this summary page of multiple US models.
Issues arise when dates provided by models based on past data are taken as fact. It is important to remember that models do not necessarily show the exact future, but instead demonstrate a possible situation that could arise based on what is currently known. Public adherence to policies such a social distancing or mask wearing can greatly influence the spread of a disease and therefore may change the predicted pandemic trajectory.
Talking Techniques | Big data and COVID-19 part 1: Facilitating and using collaborative, open data
In the first of two episodes on the role of big data in fighting COVID-19, BioTechniques Digital Editor Tristan Free speaks to Guy Cochrane about the challenges and importance of compiling and presenting the huge amount of data created regarding COVID-19.
Track and trace: monitoring the movement of communities
In response to the lockdowns and Shelter-in-Place recommendations, Google (CA, USA) created the COVID-19 Community Mobility Reports. Utilizing the technology created for Google Maps, they aimed to provide insight into how well people have responded to such policies by tracking movement trends of communities. The reports were broken down by location and demonstrate mobility changes in a region, such as a reduction in people using public transport or going to retail centers.
The data used is unidentifiable and follows all of Google’s privacy protocols; however, it marks one of the largest tracking projects to date. And many other companies and governments worldwide have followed suit, tapping the location data of millions of cell phone users in order to examine how well the world responded to lockdown orders and to visualize social distancing measures in place. It has been estimated that approximately 9.8 billion cell phones, 2200 satellites and over 25 billion digital sensors have been used to collect data worldwide, together documenting the radical shift in behavior and movement patterns that occurred as a result of COVID-19.
The large-scale tracking of movement has a larger goal, namely tracing the spread of the virus and hopefully containing it. Governments worldwide have launched track and trace apps that identify those who may have been in contact with someone who is infected, and tech giants Google and Apple (CA, USA) have combined forces to develop a Bluetooth-dependent contact-tracing framework that creates links between phones that have been in close proximity.
Going against the majority of Europe, the UK rejected the collaboration’s creation, opting instead for a centralized approach whereby contact matches will be identified by a server. Their decision received scrutiny from many as the way such an approach uses the data may not provide the sufficient privacy required. As all types of contact-tracing apps require the extensive collection and use of personal data, how this data is processed is of utmost importance and maintaining the privacy of the individual is key.
Maintaining accuracy in COVID-19 data
Predictions and reports are only as good as the data behind them, therefore ensuring that data is as accurate as possible is vital. When evaluating data about the results of diagnostic tests, the potential for false negatives or false positives should be accounted for and differences in reporting practices from different locations should be factored in.
A study recently published in mSystems found that differences in testing practices and reporting of data have resulted in regular oscillations in case numbers, creating a pattern of peaks and troughs that repeats on a near-weekly basis [1].
Looking at US national data, the team found a 7-day cycle in the rise and fall of national cases, with the patterns of oscillations matching past research that has found mortality rate is higher at the end of the week or weekend. When analyzing city-specific data, they found the cycle was slightly reduced in some areas, with a 6.8-day cycle and a 6.9-day cycle in New York City (NY, USA) and Los Angeles (CA, USA), respectively.
Despite earlier suggestions that the pattern could be related to behavior patterns and patients receiving a lower quality of care later in the week, the research team discounted societal practices as a factor as the time from exposure to symptoms starting can vary between 4 and 14 days. While these may influence outcomes, they were not found to significantly contribute to the patterns seen in the data.
When looking at the specific reporting practices they found that national data published a COVID-19-related death when it was reported, not when it occurred. In comparison, the city-specific data from New York City and Los Angeles publishes the death on the day it occurred. In larger data sets where the death is listed as the day it occurred rather than the day it was reported, these oscillations vanish.
“The practice of acquiring data is as important at times as the data itself,” commented study author Aviv Bergman (Albert Einstein College of Medicine, NY, USA). “As long as there are infected people, these oscillations, due to fluctuations in the number of tests administered and reporting, will always be observed, even if the number of cases drops.”
Similar oscillation patterns can be found in new case data and can also be explained by discrepancies in the reporting of cases. The authors stress that this should be taken into account when developing epidemiological models that are based upon reports of COVID-19 deaths or new cases.
Lab-made mimic allows for safer study of SARS-CoV-2
A lab-made hybrid virus that mimics the infectious properties of SARS-CoV-2 without the risk of human transmission could allow more researchers to join the fight against COVID-19.
Making up for missing data
Despite the huge amount of data generated during the pandemic, there are still clear gaps. Regions with easy access to healthcare and adequate numbers of testing sites have allowed researchers in those areas to accurately portray how COVID-19 has affected their city and its population. However, not everywhere has such facilities.
Even as testing infrastructure increases throughout the USA, low-income and minority neighborhoods are still behind in numbers – despite suggestions that they have been disproportionally affected by the disease – and approximately half of the reported COVID-19 cases are missing the race and ethnicity information of the patient.
Without having a fully representative dataset for the population, epidemiologists have a hole in their research – particularly if studying the racial inequality of COVID-19. Without knowing the ethnicity or race of the confirmed cases, you cannot determine the source of the high death rates, whether they are due to increased exposure, increased susceptibility to the virus or a later stage of diagnosis.
To address this, on 4 June, the US Department of Health and Human Services (DC, USA) announced that, from 1 August, laboratories will be required to report all of the patient’s demographics, including race, ethnicity, sex and age. “High-quality data is at the core of any effective public health response, and standardized, comprehensive reporting of testing information will give our public health experts better data to guide decisions at all levels throughout the crisis,” commented the Health Department’s Secretary Alex Azar. Though a positive step from the US Government, it is not clear how this will be enforced as the fields for demographic information was already on the forms for reporting COVID-19 cases – they were just left blank.
Some research groups have found ways to work around the COVID-19 data gaps, creating a “health equity interactive dashboard” that combines case and death counts from across the USA with recent government records and census data. By comparing the different data sets, correlations can be found between regions with a high death count and regions with a large African American population, as well as between regions with many confirmed cases and areas with large Latino populations. Though helping to give an insight into such correlations, the mash-up of government records and COVID-19 data can only tell us so much, and causality cannot be determined.
To truly understand the virus that is currently causing havoc globally, these gaps in data need to be addressed and a more complete dataset used for the analysis of COVID-19.