To find what is unique, stop averaging
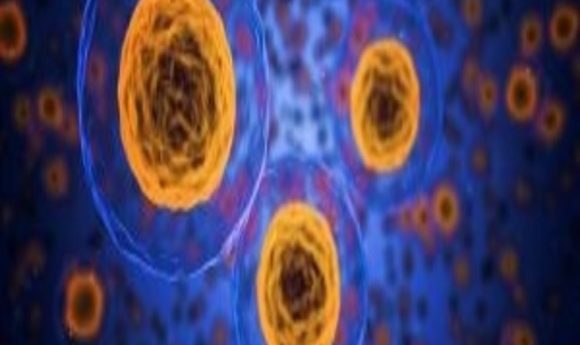
Will recent advances in single cell analysis methods help us better understand and improve human health?
Humans run at an average speed of about 10 mph over short distances. This number differs when we factor in additional parameters such as age and gender. Narrowing down to the individual level, we know that Usain Bolt holds the world record for running at an average speed of about 23 mph, which is very different from the average human speed. While averaging provides some basic information, it clearly masks some unique individual properties.
The same situation arises in cell biology research; for a long time, researchers believed that clonal cells, all of which possess the same DNA, were identical. However, around the beginning of the 21th century, evidence of varying gene expression patterns and protein profiles began to emerge among clonal cell populations; genetically identical cells were actually phenotypically heterogeneous. This implied that traditional methods relying on averages of cell populations could obscure both positive alterations that help cells adapt to their fluctuating environments and negative effects that influence disease states.
To address this heterogeneity, researchers turned to developing methods for single cell analysis. Over the past few decades, technical advances in this field have enabled analysis of thousands of single cells, and single cell profiling can now be accomplished at the genomic, transcriptomic, or protein level. When analyzing the whole genomic sequence, for instance, researchers found that cells within a tumor environment were highly heterogeneous, providing new insights into clonal cell evolution [1]. While whole genome sequencing is highly informative, it is also expensive. mRNA offers a direct window into gene expression, which eventually influences phenotype, so many researchers have focused on transcriptome analysis as a cost-effective alternative.
FISH-ing for RNA
One of the most popular methods for detecting mRNA in cells is fluorescence in situ hybridization (FISH), wherein fluorescent probes attach to complementary specific target mRNAs of interest. Each probe generally bears multiple fluorophores essential for a strong detection signal, and the intensity of fluorescence can provide a quantitative estimate of the specific transcript levels.
“One of the big limiting factors of FISH, as robust as it is, is that in most FISH protocols that people perform, they are trying to use 30 to 50 probes to target a particular mRNA of interest,” said Gable Wadsworth, a graduate student researcher at the Georgia Institute of Technology. Based on the space constraints for multiple probe attachment, only target mRNAs longer than 200 nucleotides can be detected using this method.
Wadsworth and his principal investigator Harold Kim wondered if they could develop an alternative method for detecting smaller mRNA fragments. They designed a short 26-nucleotide probe bearing a single fluorophore for mRNA detection [2]. However, the fluorescent signal from a single fluorophore was difficult to detect using a regular epifluorescence microscope.
Kim’s team overcame this issue by using an optimized combination of methanol fixation and a microscopy method wherein an inclined sheet of light is incident upon the sample. The light sheet passing through different planes enabled the team to successfully detect short transcripts in budding yeast.
Compared to the conventional FISH method that uses multiple probes, this method detects only 50% of the RNA. “With our method, we compromise the capability to detect everything, but the advantage is that we can detect much shorter RNA molecules,” noted Kim. Their method is especially useful for applications that do not require highly sensitive detection, such as relative quantification of a specific gene transcript under different conditions or determination of transcriptional heterogeneity in a clonal cell cluster. Moreover, Wadsworth estimates their method to be about 10 times cheaper than traditional FISH.
Currently, Kim and Wadsworth are also extending their single probe method to detect circular RNAs. Expression levels of circular RNAs have been shown to fluctuate in diseases such as cancer, but researchers can detect circular RNA using conventional FISH techniques only if the RNA is long enough to have 30-50 probes, said Wadsworth, who presented their preliminary findings at the Biophysical Society Meeting in San Francisco [3]. In this project, Wadsworth is combining hybridization chain reaction (HCR) and single probe mRNA detection methods to amplify circular RNA signal. “Rather than a single probe, I am using two probes that are paired. Both sit in the solution and won’t amplify, but when you mix with the target, one will open up and allow amplification to proceed,” he explained.
While RNA detection is one aspect of transcriptome analysis, another aspect is single cell RNA sequencing. “If you want as much information as possible then single cell RNA sequencing would be the best. You could get the whole transcriptome,” said Kim.
Decoding the RNA Code
Traditionally, researchers focused on sequencing coding RNAs in single cells. However, non-coding RNAs are also involved in biological processes such as transcriptional regulation and disease progression. When Itoshi Nikaido, group leader at the RIKEN Advanced Center for Computing and Communication/Center for Developmental Biology in Japan, attempted to detect non-coding RNAs using existing single cell RNA sequencing techniques, his team failed to capture them.
Most methods require pre-amplifying cDNA with reverse transcription primers and adding an adapter sequence for amplification, but reverse transcription efficiency significantly decreases when the adapter sequence is added to the primers, explained Nikaido. “So I have developed a novel method to amplify cDNA without adding an adapter sequence to the random primers, which is named ‘random displacement amplification with reverse transcription (RT-RamDA),’” said Nikaido, who recently published these findings in Nature Communications [4].
The novelty of this method is that it successfully covered a full-length RNA sequence with more than 20 kilobases, a vast improvement over existing methods, which could not sequence genes over 10 kb in size.
“RamDA sequence attempts to expand the number of detected genes and capture various kinds of RNAs, which is a completely different direction from other conventional methods for single-cell RNA-sequencing,” added Nikaido.
Advances in sequencing methods necessitate development of up-to-date analysis tools. For instance, in a dynamic process like embryonic development, cell populations differentiate into different types, explained Rodrigo Gonzalo Parra from the Max Plank Institute of Biophysical Chemistry, Goettingen, Germany. Most existing analysis tools only work if the sequencing data is from two or three different cell types.
Parra recently developed a new tool, MERLoT, which improves reconstruction of the lineage tree with increasing numbers of cell types [5]. “Our tool is good because of the algorithm we use for detecting end points and branch points and how they are connected. Most methods are not good at detecting end and branch points [of lineage trees],” said Parra.
Recently, Parra also helped develop another tool, PROSSTT, to simulate data sets for testing different analysis tools [6]. This is useful, as currently there are not many real data sets with different cell types for this purpose. “Prost makes simulations that we use to test MERLoT against different methods,” said Parra.
The Future of Single Cell Analysis
Although single cell analysis allows researchers to follow different steps of a process and reconstruct the dynamic history of how gene expression changes, it is also noisy, said Parra. In the future, these techniques will measure thousands of cells in a single experiment to help overcome the noise.
Kim seconds this opinion, specifying that the field is heading towards conducting FISH experiments on a very large scale since it is a cost-effective option that focuses on few RNA molecules of interest, simultaneously enabling detection of the transcript location. Using multiple colors instead of their single-color probe method would facilitate exponentially increasing the number of mRNA molecules that could be detected, according to Kim.
On the sequencing side, incredible projects such as the Human Cell Atlas (HCA) project are already underway. The HCA is an international collaborative effort that aims to create reference maps of all human cells for understanding human health. Researchers involved in this project have collected genomic data from over 250 thousand cells from donated human tissues to study the gene expression in individual cells. “Our understanding of human development will be transformed by the HDCA project, and could lead to significant advances in biology and medicine. We expect this fundamental research to deliver a wide range of important insights—from a better understanding of why miscarriages and genetic developmental disorders happen, through to understanding childhood cancers that have their root in development and the developmental pathways that cancer cells take advantage of in adults,” Sarah Teichmann, co-chair of the HCA Organizing Committee, noted on their website.
Nikaido aims to increase the number of cells that can be processed per one experiment and to develop a directional RamDA-seq. The HCA strongly depends on such techniques, according to Nikaido. “We hope that RamDA-seq contributes to making a full-length RNA atlas of human cell types and contributes to the HCA project from Japan,” he said.